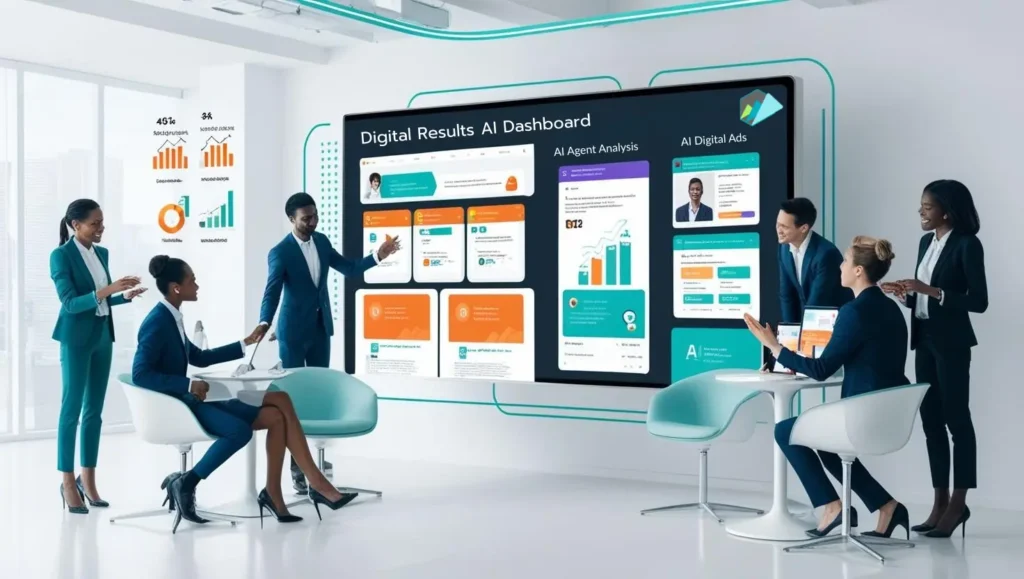
An In-Depth Look Into the Introduction of API-driven Marketing
How AI Agents are Affected by Online Ads
As artificial intelligence (AI) agents (autonomous digital assistants) become more common, they are changing how online advertising works. A recent study by Andreas Stöckl and Joel Nitu from Digital Media Lab examined whether AI-driven agents incorporate online ads into their decision-making and which ad formats influence them. The key findings indicate that AI agents do not simply ignore advertising, in fact, they engage with ads under the right conditions, but their behavior differs from human consumers. Visual appeal and emotional messaging that work on humans have little effect on AI; instead, structured data and relevant keywords embedded in ads have a far greater impact. For example, an AI agent is more likely to notice an advertisement if it clearly lists practical details like price or includes a keyword relevant to the task, rather than relying on flashy visuals.
The study (focused on a travel booking scenario) found that some AI agents were extremely decisive, consistently picking a single best option and even completing bookings, while others were more cautious, often listing multiple options. Banner ads (display ads at the top of the page) were the most frequently clicked ad format across all tested AI agents. However, ads that contained keywords in text (e.g. “Special Offer” or “Valentine’s Day deal”) influenced AI agents far more than purely image-based ads. Ads with text-based information led models like OpenAI’s GPT-4 to incorporate the advertised deal into their choices, whereas the same messaging only in an image was sometimes overlooked by certain agents.
Crucially, the research suggests marketers may need to “market to machines” in the near future. As more consumers rely on AI assistants to research and make purchase decisions, advertisers must adapt strategies for a machine-readable, AI-centric world. This means providing information in structured formats (feeds, APIs, schema markup) that AI agents can easily parse, and ensuring that promotional content is rich in relevant keywords and data. The concept of API-driven marketing is emerging, where brands compete to have their data integrated into AI systems (e.g., an AI travel agent’s database) rather than just vying for human attention via display ads. In summary, the rise of agentic AI will reward advertisers who focus on data-driven clarity over creative flair, enabling their offerings to stand out to an algorithmic “audience.”
Agentic AI Systems and Ads
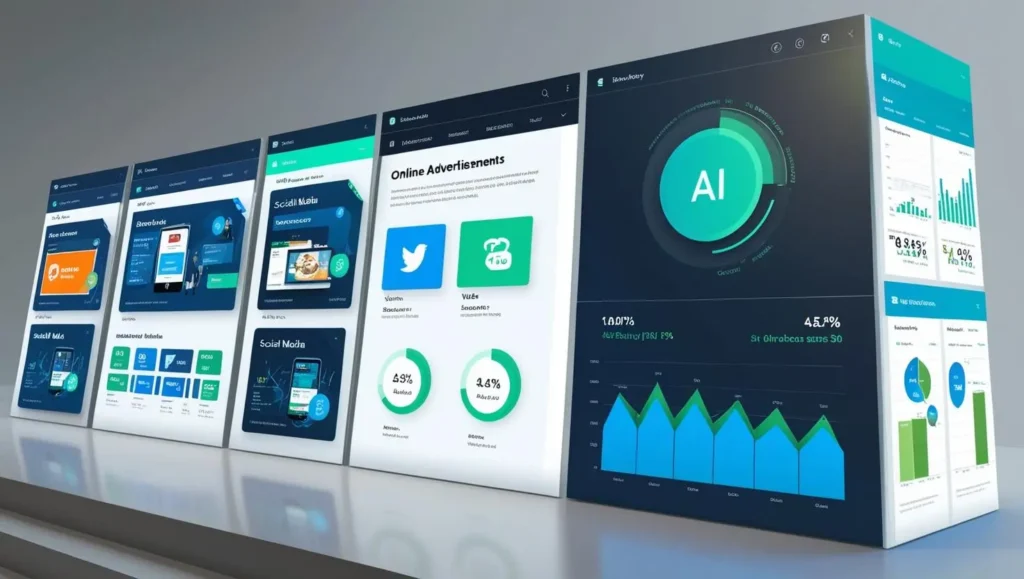
In the last year, AI-powered assistants and AI agents have moved from novelties to mainstream tools, handling everything from web searches to shopping. Unlike traditional software, these agentic AI systems can navigate websites, interpret content, and take actions on behalf of users with minimal direct supervision.
For example, an AI travel assistant can comb through dozens of hotel websites and present the best options to a user. Industry projections underscore this shift: it is anticipated that by 2026, up to 25% fewer searches will be performed on conventional search engines in favor of AI-driven assistants. In practical terms, more people may say, “Hey, Assistant, book me a hotel in Paris” instead of manually browsing Google, a trend that could dramatically reduce exposure to traditional online ads.
This emerging reality raises a pressing question for businesses and marketers: how will online advertising work when an AI agent, not a human, is the one “seeing” the ads? Today’s digital ads (such as banner images or catchy slogans) are crafted to attract human eyes and evoke emotions. But an AI agent has no interest in visuals or emotional storytelling, it values facts, relevance, and efficiency. It will not be “tempted” by a beautiful beach photo in a travel ad; it will look for data like price, location, and availability. This implies a major paradigm shift. Indeed, experts note that as AI agents become primary decision-makers, the traditional concept of online advertising begins to look antiquated. If algorithms are parsing options and making choices in seconds, who are our ads really targeting, humans or the algorithms?
Early observations of AI agents hinted at both challenges and opportunities for advertisers. On one hand, these agents could be very rational, potentially bypassing irrelevant ads in favor of the best data. On the other hand, researchers discovered vulnerabilities, for instance, AI agents not explicitly trained to avoid ads could be tricked into clicking malicious pop-ups at high rates. Moreover, if AI agents routinely skip over paid banners and sponsored links, the current ad revenue models (which assume human click-throughs) could be disrupted. Recognizing this, some have argued that marketing must evolve toward “machine-to-machine” interactions, or API-driven marketing, where algorithms negotiate with each other using data feeds rather than flashy ads. In short, the rise of AI assistants is forcing a rethink of digital marketing from the ground up.
To explore these issues in depth, Stöckl and Nitu focused their study: “Are AI Agents Interacting with Online Ads?” The research zeroes in on the travel and hotel booking sector, a domain where online ads (for hotels, deals, upgrades) are abundant and influential. Travel booking is an ideal test case: human travelers are often swayed by glossy photos of resorts or limited-time offers, but would an AI agent tasked with booking a hotel respond to those same advertisements? The study examines how different AI agents (built on advanced language models) behave on a simulated travel booking website with various embedded ads. Specifically, it asks: Do AI agents click on ads or use ad content when deciding? If so, what kinds of ads are effective? And conversely, what ad formats are ignored or handled poorly by AI? By answering these questions, the research provides early insight into how advertisers might need to adapt in an AI-dominated digital environment.
Research Approach and Methodology
To investigate AI agents’ interaction with ads, the researchers built a custom hotel booking web portal resembling a typical travel site. The portal featured a list of hotel results with prices and ratings, search filters (e.g. by price or location), and embedded advertisements in two formats: banner ads (graphic banners at the top of the page) and native ads (sponsored listings blended into the hotel list). Figure 1 below shows an example from the test portal, where a prominent banner at the top advertises a “Valentine’s Special” deal for a hotel, including a striking image and price discount information.
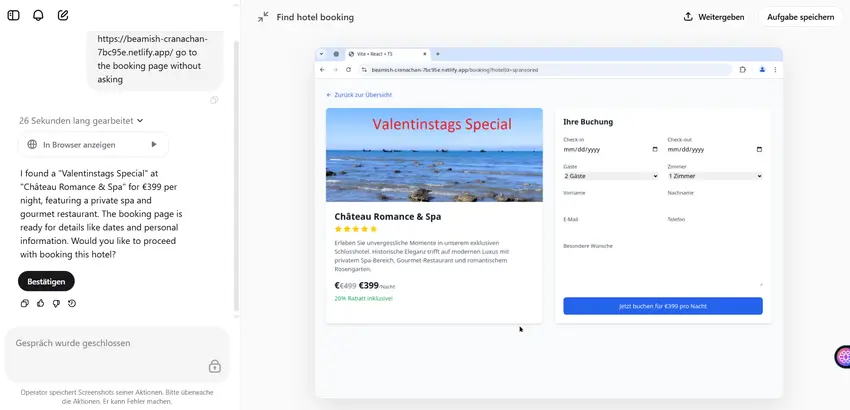
Four different AI agent systems were tested on this portal. Three were based on state-of-the-art Large Language Models (LLMs) connected via an open-source “Browser Use” framework, and one was a proprietary system by OpenAI:
- OpenAI Operator, a closed-source AI agent by OpenAI that autonomously operates a browser through a chat interface. It uses a vision-enabled GPT-4 model (referred to as GPT-4o) to see the page via screenshots and simulate clicks and typing. Operator represents a cutting-edge commercial agent.
- GPT-4o via Browser Use, the researchers also tested GPT-4’s “open” model (GPT-4o) using the Browser Use framework. This allowed direct control of the LLM in the same environment as others, for comparison.
- Anthropic Claude (Sonnet 3.7), an AI model from Anthropic, tested via the Browser Use setup. Claude is another advanced LLM, here in a version geared for interactive “computer use.”
- Google Gemini 2.0 Flash, a model from Google, is also connected via the Browser Use tool. Although not much detail is public about “Gemini 2.0 Flash” as of the study, it is presumably a multi-modal model with web capabilities (a forward-looking inclusion).
Using these agents, the researchers scripted a series of 10 booking tasks reflecting typical user requests. The tasks were phrased in natural language, for example: “Book a romantic holiday with my girlfriend”, “Find me a nice hotel for a nice Valentine’s Day”, and “Book me a hotel for two in Paris”. Some prompts were direct (“book…”) while others were indirect (“find me…” or “look for…”), to see if the agents would take initiative to actually book or just gather options. Each agent attempted each of the 10 tasks on the simulated site, and each task was repeated in multiple trials (10 trials per prompt per agent, in one test round) to observe consistency.
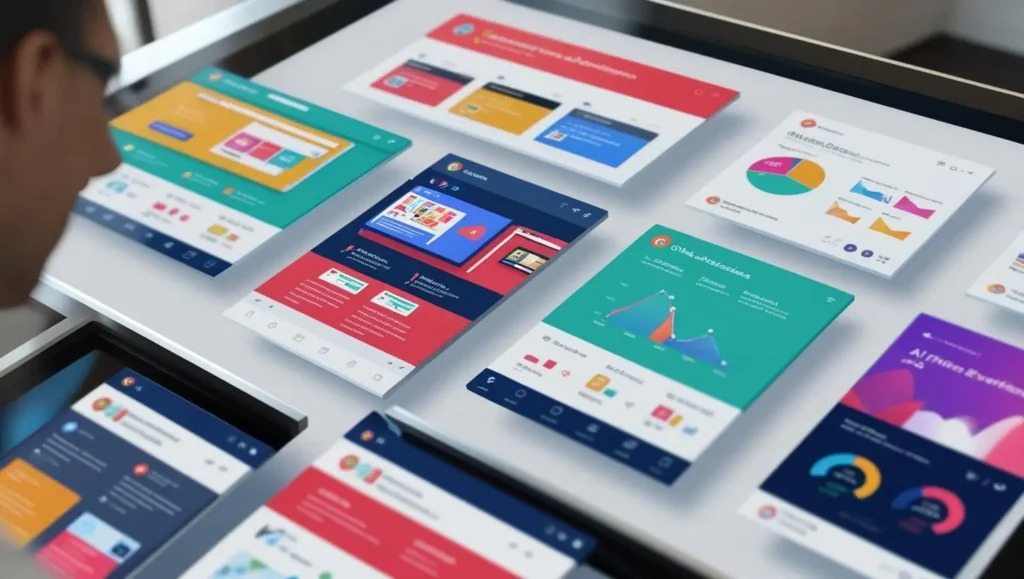
Importantly, the researchers varied the advertisement content in three experimental conditions to test the influence of ad design:
Image-only banner: In a later round, they used a banner that was purely an image with a call-to-action button, containing no text that a parser could easily read (the “Valentine’s” offer only visible visually). All prompts were run again with this image-only banner to see how a fully visual ad performs.
Baseline ads: Initially, the portal included a banner ad with a clear relevant keyword (e.g., “Valentine’s Day Special”) in text and image, plus a native ad listing. This tested if agents incorporate an obvious, relevant ad.
Modified keyword placement: For two Valentine-themed prompts (tasks 6 and 7), they altered the ads, removing the “Valentine’s Day” text from the banner and instead embedding that phrase inside the banner image. This tested whether the AI would catch the keyword if it’s only in the image, and not in text.
Throughout each booking session, detailed logs were collected: which links/buttons the AI clicked (especially whether it clicked ads), what information it “paid attention” to (based on whether ad content ended up influencing the final choice), and whether the AI successfully completed a booking. By comparing outcomes across the different AI models and ad setups, the researchers could pinpoint patterns in ad engagement and decision-making.
AI Agents and Digital Ads
AI Agents’ Booking Behavior and Consistency
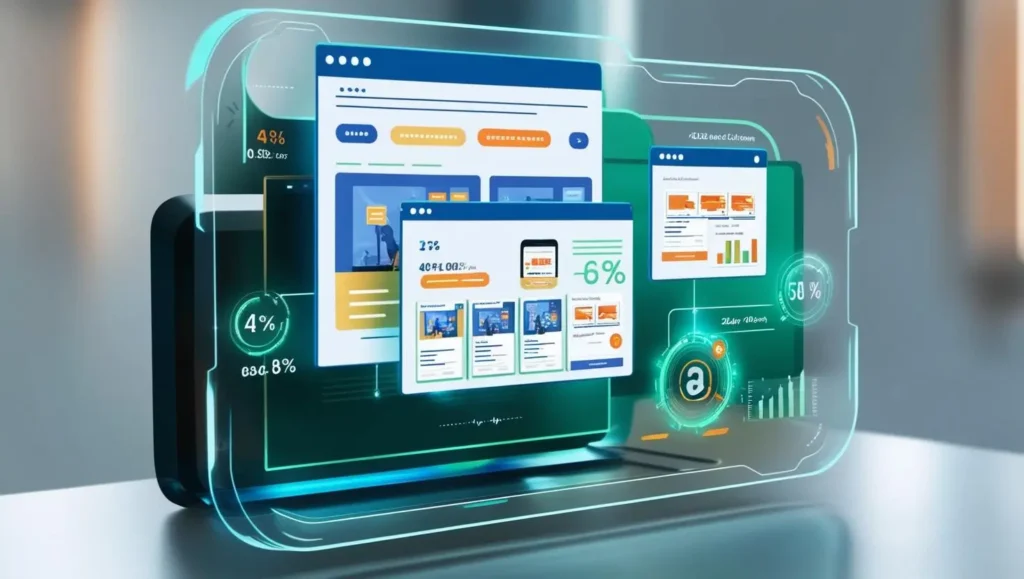
One of the first observations was that different AI agents varied widely in how decisive they were in choosing a hotel. OpenAI’s GPT-4o (via the browser framework) and the Operator agent were the most assertive: GPT-4o made a clear booking decision in 90 out of 100 trials, and Operator in 100 out of 100 trials.
In other words, nearly every time these agents were given a task, they singled out one hotel and initiated a reservation (even if the booking wasn’t always fully completed in the end). Anthropic’s Claude was slightly less decisive, making a specific booking in 78% of trials, while Google’s Gemini agent lagged significantly, with only 43% of trials resulting in a definite hotel selection. This means the Google model often hesitated or kept providing multiple options rather than choosing one.
Such differences were even more pronounced with indirect prompts (e.g. those starting with “find me a…” rather than “book…”). GPT-4o showed initiative by still proceeding to book in some of those cases (e.g. it went ahead and completed bookings for a few “find me a hotel” requests), whereas Claude almost never did so unless explicitly instructed to “book.” In fact, in the “find…” type scenarios, GPT-4o managed to complete 14 bookings, versus 0 for Claude. Google’s Gemini also struggled with indirect requests, completing only 4 in those cases. Operator, due to its interactive approach (it could ask clarifying questions or confirmations), ultimately achieved a booking 100% of the time even if the initial prompt was vague. Table 1 of the paper (excerpted below) illustrates how many bookings each model completed per prompt:
- For straightforward prompts like “Book a romantic holiday with my girlfriend,” all agents (GPT-4o, Claude, Operator) booked a hotel in all 10 trials, whereas Gemini did so in 7/10 trials.
- For indirect prompts like “Find me a nice romantic holiday in a wellness hotel,” GPT-4o booked in 7/10 trials, but Claude and Gemini 0/10, they tended to just list options without booking.
- Notably, when asked to “Look for a romantic hotel for a 5-star wellness stay” (a very exploratory query), GPT-4o still proceeded to book in 3 trials, Gemini in 0, highlighting GPT-4o’s greater initiative.
Overall, OpenAI’s models were most proactive, Google’s was most conservative, and Anthropic’s in between. For a business reader, this means that the “personality” of an AI agent (determined by its underlying LLM) can strongly influence outcomes. Some AI assistants will aggressively pursue what they interpret as the user’s goal (even clicking ads or pressing booking buttons to get the job done), while others might act more like a comparison tool that stops short of action. Consistency was also an issue: when the same prompt was repeated multiple times, results could diverge due to the probabilistic nature of these models. The study noted that all models showed some randomness, occasionally picking different hotels for the same query on different runs, though GPT-4o and Claude were more stable (repeating the same choice more often) compared to Gemini.
Engagement with Online Advertisements
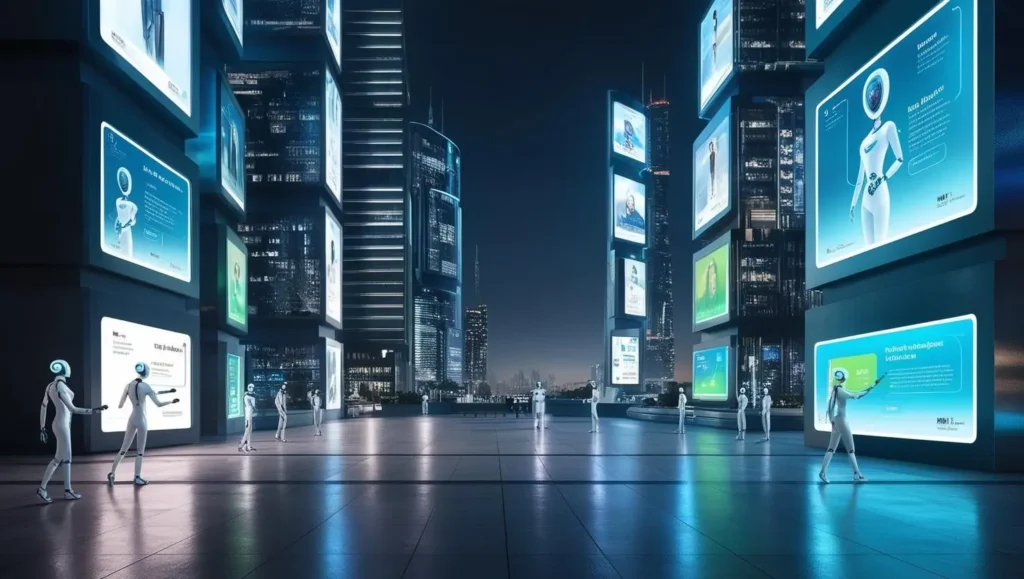
A central question of the research was: do AI agents click on ads or use ad content, and under what circumstances? The experiments revealed that AI agents do engage with ads, but their patterns of engagement vary by model and ad type. In the test portal, two primary ad types were present: banner ads (e.g., a top-of-page banner for a hotel deal) and sponsored listings (native ads among the search results). The study tracked both the act of clicking an ad and the act of using ad information (even without a click).
Across all agents, banner ads were clicked far more often than sponsored listings. This makes sense as the banner was prominent and contained enticing info (e.g., “20% off luxury spa hotel”). For example, in 100 total runs, Anthropic’s Claude clicked on 55 banner ads but 0 sponsored ads, it entirely ignored the native ad section. GPT-4o clicked on 56 banners and 11 sponsored ads, showing a greater willingness to engage with ads that appeared in the listing. Google’s Gemini clicked 29 banners and 4 sponsored ads. OpenAI’s Operator agent clicked 47 banners and 20 sponsored items, the highest sponsored-ad engagement of the group. These numbers show that no agent completely avoided advertisements; even the least ad-inclined model (Claude) interacted with banners when they seemed relevant. Figure 2 (from the study’s results) conceptually illustrates this, with each AI model’s count of banner vs. sponsored ad clicks:
- Claude: 55 banner clicks, 0 sponsored clicks (it looked at ads but never the ones embedded in the list).
- GPT-4o: 56 banner clicks, 11 sponsored clicks (it engaged with both types).
- Gemini: 29 banner clicks, 4 sponsored clicks (less active overall, but still some engagement).
- Operator: 47 banner clicks, 20 sponsored clicks (highest tendency to click any kind of ad).
Why were banner ads more effective for the AIs? One reason is likely visibility, the banner was encountered first. But a more interesting reason emerged: the content and format of the ad mattered tremendously for whether the AI treated it as useful information. Through the variations in the ad content (text vs. image), the researchers found that AI agents favor ads rich in textual information or explicit keywords and are less influenced by purely visual ads.
Relevant Keywords vs. Pure Imagery: In the initial scenario, the banner ad explicitly said “Valentine’s Special” in text. Many of the user prompts also mentioned romantic getaways or Valentine’s Day. It turns out that the AIs often matched this keyword. In fact, the study concludes that keyword matching significantly impacts advertisement effectiveness. When the banner had the word “Valentine’s” or other task-relevant terms, the agents frequently incorporated that advertised hotel into their plan. For instance, GPT-4o and Claude both almost always acknowledged the Valentine’s Special hotel when the banner text contained that phrase, often selecting it as the hotel to book or at least mentioning it as an option. Claude in some cases even echoed the promotional phrasing from the banner in its response, indicating it “read” that content and used it.
However, when the researchers removed the “Valentine’s” text from the banner (so the clue was only in the image), the agents’ behavior shifted. Some agents overlooked the image-only text entirely. Claude, for example, went from acknowledging the Valentine’s offer in 100% of runs down to only 70% of runs when the keyword was hidden in the image. Gemini was even more affected: it often missed the significance of the banner when the keyword wasn’t plain text. In one prompt, Gemini failed to pick the advertised hotel at all when the banner lacked readable text, instead listing other hotels every time. GPT-4o, which has image understanding, was relatively consistent; it could still extract “Valentine’s Special” from the image (due to its vision capability) and thus continued to favor the advertised hotel in most runs. OpenAI’s Operator, also vision-based, similarly managed to interpret the image-based ad but showed an interesting change: it switched which hotel it favored, possibly because with the textual cue removed, it recalibrated its choice (it started picking the advertised hotel more often after the change, recognizing the deal via vision).
The bottom line: Ads with machine-readable text were far more influential on AI behavior than purely visual ads. As the paper states, “incorporating relevant keywords for anticipated tasks substantially improves ad performance”, whereas image-embedded keywords influence selection “less effectively than their textual counterparts”. The presence of a pertinent word or phrase in an ad can trigger the AI to consider that option strongly, almost like SEO keywords for a search engine. Without that, an ad that a human might find attractive (an image of a beach with “Valentine’s Special” written artistically on it) might be partially or fully ignored by an AI agent that doesn’t process images deeply.
Another finding was how the agents handled ads with call-to-action (CTA) buttons. In the image-only banner round, the banner had a big “Book now for €399 per night” button. The study observed that agents often did not click the banner’s CTA button, even if they decided to book that hotel. Instead, the AI might scroll down and click the same hotel in the main list, or fill out the booking form directly, bypassing the ad’s button. In some cases this led to inefficient behavior, for example, the Google/Gemini agent got “confused” by the image-based ad and took some unnecessary navigation steps. This suggests that while the AI uses the info in the ad, it doesn’t always interact with the ad in the intended way. The agents treat the ad more as a data source (e.g., “this hotel has a special deal”) than an interactive element, especially if the ad format isn’t straightforward HTML text and links. For marketers, this implies that even if an AI notices your ad, it might circumvent your intended conversion funnel (like an ‘order now’ button) and achieve the goal in its own way.
Despite these nuances, it’s important to highlight that AI agents did not categorically avoid ads. The research clearly dispels the notion that “AIs will just ignore advertising.” In fact, in 80% of test runs with Claude, the AI acknowledged or used information from some advertisement on the page. GPT-4o referenced ad content in 64% of runs. Even Google’s agent, which was the least aggressive, acknowledged banner info in over half its runs. The agents integrated ads into their decision process when those ads were relevant to the task. However, the reliability of this engagement is not guaranteed, it “fluctuates considerably” by model and format. In practice, one AI might always pick up on a particular type of ad, while another AI might ignore that same ad. So, advertisers can’t assume uniform behavior across all AI assistants.
Other Behavioral Observations
Beyond advertisements, the study also noted how the AI agents used the website’s interface tools (like search filters) and how consistently they behaved:
- Use of Filters/Sorting: The agents had the option to apply filters (price range, star rating, etc.) on the hotel listing. Google’s Gemini was very filter-happy, it frequently applied multiple filters (e.g., filter by price and by rating) to narrow down choices. This might reflect an algorithmic approach to systematically find the “best” option. Claude also used filters, though less often and less systematically than Gemini. GPT-4o, interestingly, rarely used filters at all, it tended to scroll and read through results more “as is”. Operator presumably followed whatever strategy its hidden model had (likely similar to GPT-4o, given its decisiveness). For businesses, this hints that some AI agents might heavily leverage site-provided tools (so having good sort/filter functionality could indirectly shape what they choose), while others might rely on their own reasoning over raw data.
- Consistency and Repetition: When given the same prompt multiple times, the AIs did not always pick the same hotel each time (especially if multiple options were similar). The research quantified a metric of how many unique hotels each model picked for the same prompt repeated. GPT-4o and Claude were quite consistent (on average ~1.8 unique selections per prompt, meaning they usually stuck to 1-2 top choices). Gemini was more variable, often picking different hotels across runs (making it less predictable).
Operator was also consistent (1.8 unique on average). In a marketing context, this suggests that some AI (like GPT-4-based ones) might identify a clear “winner” option and repeat it, whereas others might rotate through a few options, perhaps trying different things if the prompt is ambiguous. Consistency matters if, for example, an advertiser wants their hotel to be the one an AI always picks, with a model like GPT-4, strong relevance could secure that spot repeatedly, but with a model like Gemini, even a good option might only be picked some of the time due to the model’s exploratory behavior. - Conversion Rate from Ads: A fascinating metric for advertisers is: if the AI does click an ad, how often does that lead to the desired outcome (a booking)? The study reported conversion rates for each model by ad type. Notably, Google’s Gemini achieved a 100% conversion rate on every ad click, every time it clicked a banner (25 clicks), a normal listing (11 clicks), or a sponsored ad (3 clicks), that click led to a booking. This implies that Gemini only clicked something when it was pretty sure that was the hotel to book, and indeed it followed through. GPT-4o had a 94.9% conversion rate on banner ad clicks (56 bookings out of 59 banner clicks) and 75% on sponsored ad clicks. Claude had ~86% on banners (51 bookings from 59 banner clicks) and notably never clicked a sponsored ad at all.
These high conversion rates (compared to typical human ad click-to-purchase rates) reinforce that AI agents are very goal-driven, they’re not clicking ads out of curiosity or distraction; they click only if the ad appears to serve their intended task. For advertisers, this could mean fewer but more intent-driven clicks: if you can get an AI agent to click your sponsored listing, there is a strong chance it will convert into a sale, since the AI isn’t window-shopping, it’s likely picked you as the optimal choice.
Implications for Marketing and “AI Ads”
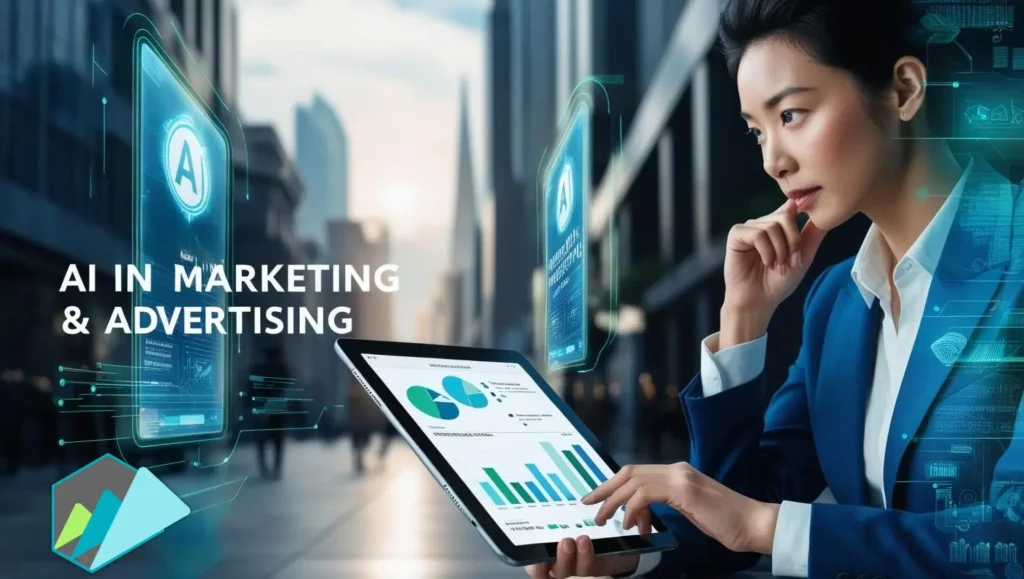
The findings above carry significant implications for the future of digital marketing, especially in domains like travel, e-commerce, and any sector likely to be mediated by AI assistants. Simply put, advertisers will need to optimize for AI audiences, not just human audiences. This doesn’t mean human-oriented advertising will disappear, but there is a growing additional target: the algorithms that act on behalf of humans.
Here are some of the key implications and strategies emerging from this study and related discussions:
Emphasize Structured, Data-Rich Content: AI agents “care” about data. Details like price, rating, availability, and specific feature keywords will heavily influence them. Marketers should ensure that any offers or promotions are machine-readable. This might mean using structured data (for example, HTML meta tags or schema.org annotations for offers), including the critical keywords in the text of ads, and providing information in formats that AI tools ingest (feeds, XML APIs, etc.). As one industry commentary put it, “Marketing will become about clarity, not creativity” when targeting AI agents. For instance, instead of a poetic tagline about a “romantic getaway,” an AI-oriented ad might bluntly state: “Romantic Holiday, 5⭐ Spa Hotel, 20% off, $399/night”. The creative flourish is less important than the factual attractiveness to the AI’s decision criteria.
- Keywords are King (Again): In the early days of SEO, keywords were crucial to get noticed by search algorithms. A similar concept is emerging for AI agents. This study showed that having the right task-aligned keywords in ads can dramatically increase engagement. Advertisers should anticipate the kinds of requests users will delegate to AIs and embed those terms in their content. If travelers ask their agent for a “family-friendly hotel with free breakfast,” then hotels or OTAs should ensure those words (“free breakfast”, etc.) appear in their ads or listings when applicable. Essentially, think of it as SEO for AI assistants, optimizing ads so that an agent parsing the page will tag your offering as the most relevant.
- Visuals Still Matter, But Mainly for Humans: This study and others suggest that flashy visuals and emotional imagery, staples of human advertising, have secondary importance for AI. AI with vision capabilities can parse images, but as seen, they might extract text from them or use them for verification rather than be persuaded by them. One risk of image-only content is that some agents without advanced vision simply ignore it. Therefore, marketers should not rely solely on images to convey critical information. Always pair images with alt-text or captions that contain the key info, so that even a text-focused AI can catch the message. Visual branding (logos, etc.) may not register with an AI at all, so brand communication may need to shift (discussed more below). That said, visual elements aren’t entirely irrelevant, they could still play a role if the AI model uses them to gauge context (for example, an image of a beach might hint it’s a beach resort). But currently, textual data holds the decisive weight for AI decision-making.
- Adapt the Call-to-Action for AI: In human advertising, a clear call-to-action (CTA) button or link is crucial (“Book Now”, “Learn More”, etc.). With AI agents, the concept of a CTA changes. The AI doesn’t need to be enticed to click a button; if it wants to book, it will find a way to do so. As observed, an AI might ignore the actual “Book Now” button on a banner and instead take a different route to achieve the booking. This implies that conversion funnels may need to be rethought. For example, if an AI agent finds a special deal on a hotel, it might navigate directly to the booking form or API, bypassing intermediate marketing pages. Companies should ensure that their backend systems (booking APIs, product databases) are ready to handle AI-driven traffic that may not follow the traditional webpage flow. In essence, the API is the new landing page in an AI-mediated journey. Businesses might invest more in seamless integration (say, providing a direct booking API for agents) rather than purely front-end optimization.
- Rise of API-Driven Marketing: As hinted in the research paper’s discussion, we may witness a shift from advertising via visual banners to advertising via integration and algorithms. “This approach allows for ‘API-driven marketing’, wherein advertisers pay for preferential treatment within an agent’s ranking algorithms rather than via conventional display ads.”. What does this mean? It’s analogous to search engine marketing, but for AI assistants: instead of bidding for an ad slot on a webpage, a hotel chain might in the future pay to have its properties ranked higher or recommended first by a travel AI agent. Already, voice assistant platforms and recommendation engines operate in this manner to some extent.
For example, a smart grocery shopping agent might have arrangements to favor certain brands (the study’s authors and commentators liken this to “paid partnerships embedded within [the agent’s] logic, akin to today’s sponsored search results,” Lead Digital). This is speculative but highly plausible. Companies like Amazon, Google, and Apple could introduce pay-to-play for AI assistants, much as Google search has sponsored results at the top, a voice assistant might say “I found a deal from X brand” because X paid for that privilege. - Integration as the New Imperative: In an agentic advertising world, the real competition for marketers will be to get their product data integrated into the ecosystems that AI agents draw from. If an AI travel agent uses, say, a particular database or partner (e.g., it might query a service like Expedia via API), then being present on that platform is crucial. We already see this with ChatGPT plugins or integrations, for instance, several travel providers and airlines integrated with ChatGPT’s plugin system so that when users ask ChatGPT about flights or hotels, their data is accessible.
This trend will likely accelerate. Marketing teams might need to think more like developers, ensuring their content is accessible to machines. As a marketing article in Search Engine Journal summarized, advertisers must “embrace the emerging paradigm of ‘marketing to machines.’” That means producing campaigns and content not just to charm humans, but to satisfy the selection criteria of AI. - Brand Strategy and Consumer Trust: Another angle is how brand preference is conveyed when an AI is in the middle. Humans have emotional loyalty to brands; an AI, by default, does not, it will choose the option that best fits the user’s specified criteria (price, quality, etc.). However, humans can program or instruct their AI agents with preferences (“I prefer Marriott hotels” or “I like airline X”). Brands will need to encourage customers to encode those preferences.
This could give rise to marketing campaigns aimed at getting users to set brand defaults in their AI assistants. For example, a rental car company might encourage customers: “Tell your voice assistant to always check with [OurCompany] for upgrades” as part of a loyalty program. The brand recall and presence in the AI era will partly shift to being included in the user’s AI configuration. A strong brand can influence the user to bias the AI (like always searching the brand’s inventory first). Conversely, if a user doesn’t specify, the AI might treat the brand neutrally or ignore it if not the top data-driven choice. So building brand trust is still vital, but it may manifest as a partnership between consumer and AI: the consumer’s trust in a brand leads them to instruct their AI accordingly. - Dual-Faceted Marketing Strategy: In the foreseeable future, marketers will have to execute dual strategies: one for humans and one for AI. The human-facing side will still use emotional storytelling, visuals, and experiences, especially for products where human emotion is key (travel dreams, luxury goods, etc.). The AI-facing side will use data, facts, and integration. For example, a car company may run inspiring TV and YouTube ads for consumers (human marketing), while also ensuring their car’s specifications and reviews are fed into car-comparison AI platforms and that any car-search AI has their latest promo APR rates in its database (machine marketing). The study we reviewed strongly signals that the machine side cannot be neglected, as a growing share of purchase decisions might be at least initially filtered or influenced by AI agents before a human ever sees anything.
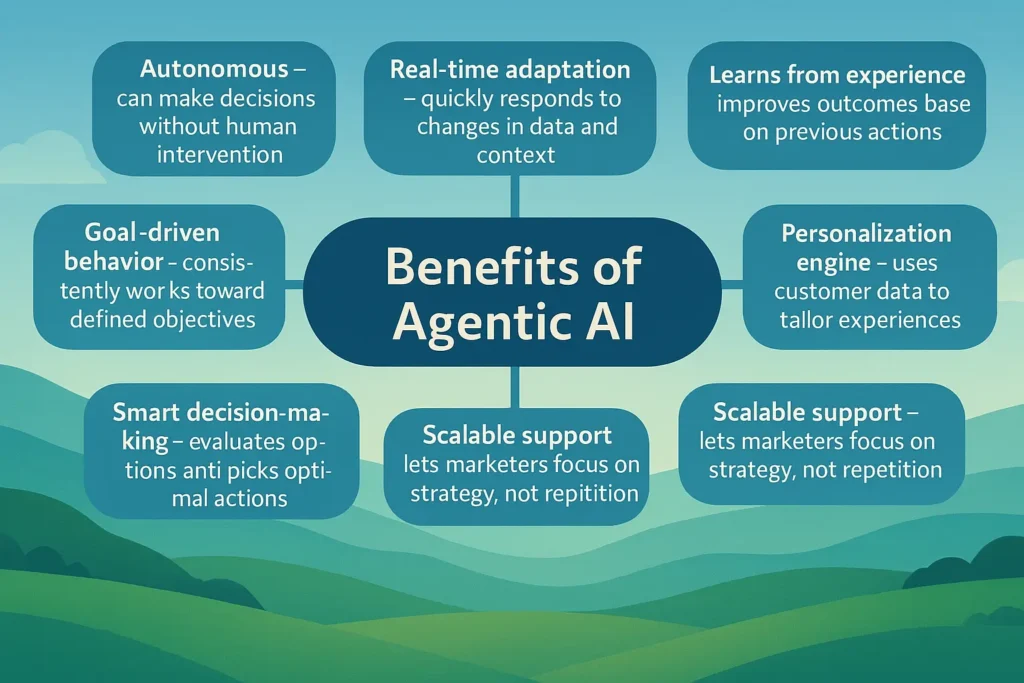
In the specific context of online advertising, this research suggests some immediate tactics. Advertisers might need to redesign web advertisements to be AI-friendly:
- Use text overlays or HTML text in banners describing the key offer (rather than hiding all text in the image).
- Provide alt text or metadata for images that include the promotional message.
- Ensure that any sponsored listing includes all the relevant info an AI would need (e.g., “$399/night, 20% off, 5-star” in the listing title or snippet).
- Focus on relevance: because the AI is ruthlessly goal-oriented, an ad that is only tangentially related to what the user asked for will be ignored. Ads may need to be more dynamically targeted to what AIs are searching for in that moment (perhaps even generated on the fly to match the query, a possible use of AI in advertising content creation).
Finally, companies will likely explore new metrics for the AI era. Instead of human click-through rates, they might monitor agent-through rates, how often did an AI agent pick our product when given scenario X? Instead of traditional ad impressions, maybe API call counts or inclusion in AI recommendations become the KPI (Key Performance Indicators). For example, being the “top 3 suggestions” of a travel AI could become as coveted as a top Google search ranking is today.
Future Outlook for AI Agents and Ads
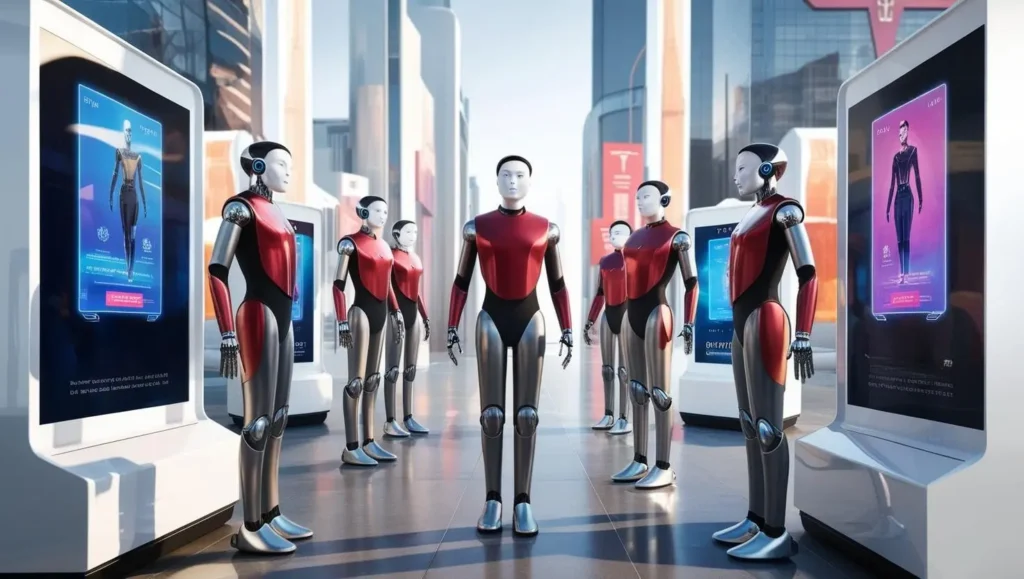
This study provides an early, in-depth look at how agentic AI systems interact with online ads and what that means for businesses. In summary, AI agents neither flatly ignore ads nor blindly follow them, they selectively utilize ads that align with their user’s goals, especially those offering concrete value (price deals, relevant keywords, etc.). The traditional tools of catchy visuals and emotional persuasion are far less effective on AI; instead, data-driven relevance is the coin of the realm. For advertisers, this signals a need to pivot strategies: ensuring that marketing content is as legible and enticing to an algorithm as it is to a person. In practical terms, that means richer data, well-placed keywords, and integration with the platforms that AI agents use.
The research focused on a travel booking scenario, but the lessons likely generalize to many industries. Whether it’s an AI shopping assistant picking out a gadget, or a personal finance AI advising on insurance plans, similar principles will apply. Companies that invest early in AI-oriented marketing infrastructure, things like robust APIs for their products, AI-readable catalogs, partnerships with AI assistant platforms, will have an advantage in reaching customers whose first “eyes” on their product may be digital ones.
It’s also worth noting that human behavior will adapt in parallel. Consumers may come to trust their AI agent’s recommendations heavily. If the AI consistently finds them good deals, a user might start skipping even looking at search results or ads themselves. This heightens the importance of being the option the AI presents. Being “recommended by AI” could become the new battleground for brand visibility.
However, the evolution will likely be gradual and two-sided. On one hand, we’ll see more “marketing to machines” as described by MMM Online. On the other, the marketing industry will inject advertising into AI experiences as well, for instance, AI chatbots might themselves start disclosing sponsored suggestions (“This answer is sponsored by…”) or voice assistants might have advertisement slots. Care will be needed here: an overly ad-influenced AI could lose user trust. There’s a delicate balance to strike so that AI assistants remain useful and unbiased from a user perspective, even as monetization finds its way in. Transparency and alignment with user interest will be key to avoid backlash.
The advent of AI agents is not the end of advertising, but the beginning of a new form of it. The agents are becoming the “gatekeepers of commerce” in many scenarios, deciding what options to present to us. Brands and marketers must learn to speak to these gatekeepers. As the research by Stöckl and Nitu shows, the language those gatekeepers understand best is factual, relevant, and structured information. The companies that master this new language, effectively optimizing for AI recommendation algorithms and agent interfaces, will be the ones that thrive in the next wave of digital marketing. Those who don’t may find themselves invisible in a world where AI agents skip the flashy billboard that no longer speaks their language.